AI for a sustainable chemistry
Drug design, sustainable energy storage, and chemical production are just some of the challenges in chemistry where AI can find applications. Kjell Jorner, the new Professor of Digital Chemistry at the Institute for Chemical and Bioengineering at the D-CHAB, wants to drive this development and accelerate the transition to sustainable chemical production in the field of catalysis.
Kjell Jorner prefers analog activities when he needs to free his mind to solve digital challenges: A native of Sweden, he is an avid runner, a budding raclette enthusiast, and a coffee connoisseur who owns “too many” coffeemakers, including Bialetti (his favorite). On the day of the interview, he also offers coffee in his offices, which also serve as a virtual laboratory. From here, Kjell Jorner and his team explore the molecular world using artificial intelligence, which must be guided with both data and knowledge before it can provide answers. Artificial intelligence (AI) is a patient student and – it is hoped – part of the solution to pressing problems in chemistry.
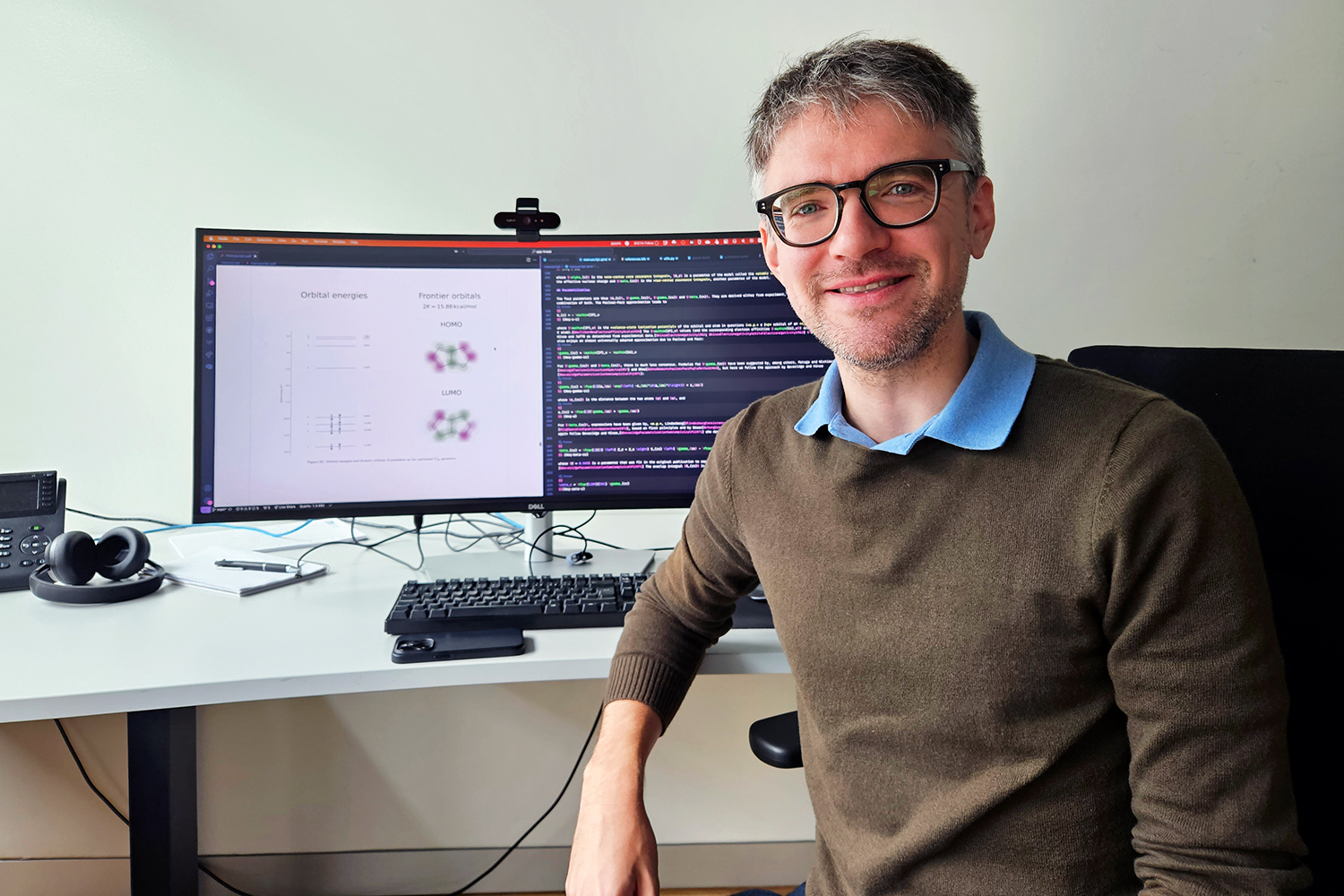
Indeed, computational research can be used to explore questions that are difficult or impossible to study in the laboratory: Predictions for things that would be experimentally unfeasible or too expensive and time-consuming. "Accelerating the discovery process is a major goal of computational methods and artificial intelligence," says Jorner, "for example, the time from identifying a target to bringing a drug to market can be significantly reduced. The same is true for reactivity and catalysis, my area of expertise. With the methods that we are developing, AI could allow us to find new catalysts much faster."
When the learning material is missing
Initially, Jorner focused on physical organic chemistry at the University of Uppsala. As a Ph.D. student, he studied the photochemistry of organic compounds in the lab. "However, I soon drifted into simulation. I enjoyed working on the computer and getting quick results. By doing tests with simulations, you can save yourself from synthesizing molecules that do not have the desired properties and focus instead on the more promising ones."
It sounds easier than it is. In chemistry, modeling, especially with the help of AI, is a big challenge. You have to understand: AI is a multifaceted umbrella term. It includes expert systems (e.g., mechanistic models based on chemical theories), which are intelligent and make predictions according to predefined rules but do not learn from data, and it includes machine learning (ML), the dominant approach today, where the system learns from as much data as possible and, on this basis, makes its own rules for making predictions (e.g., for chemical reactions). ML also includes Deep Learning – artificial neural networks with input, output, and many hidden layers.
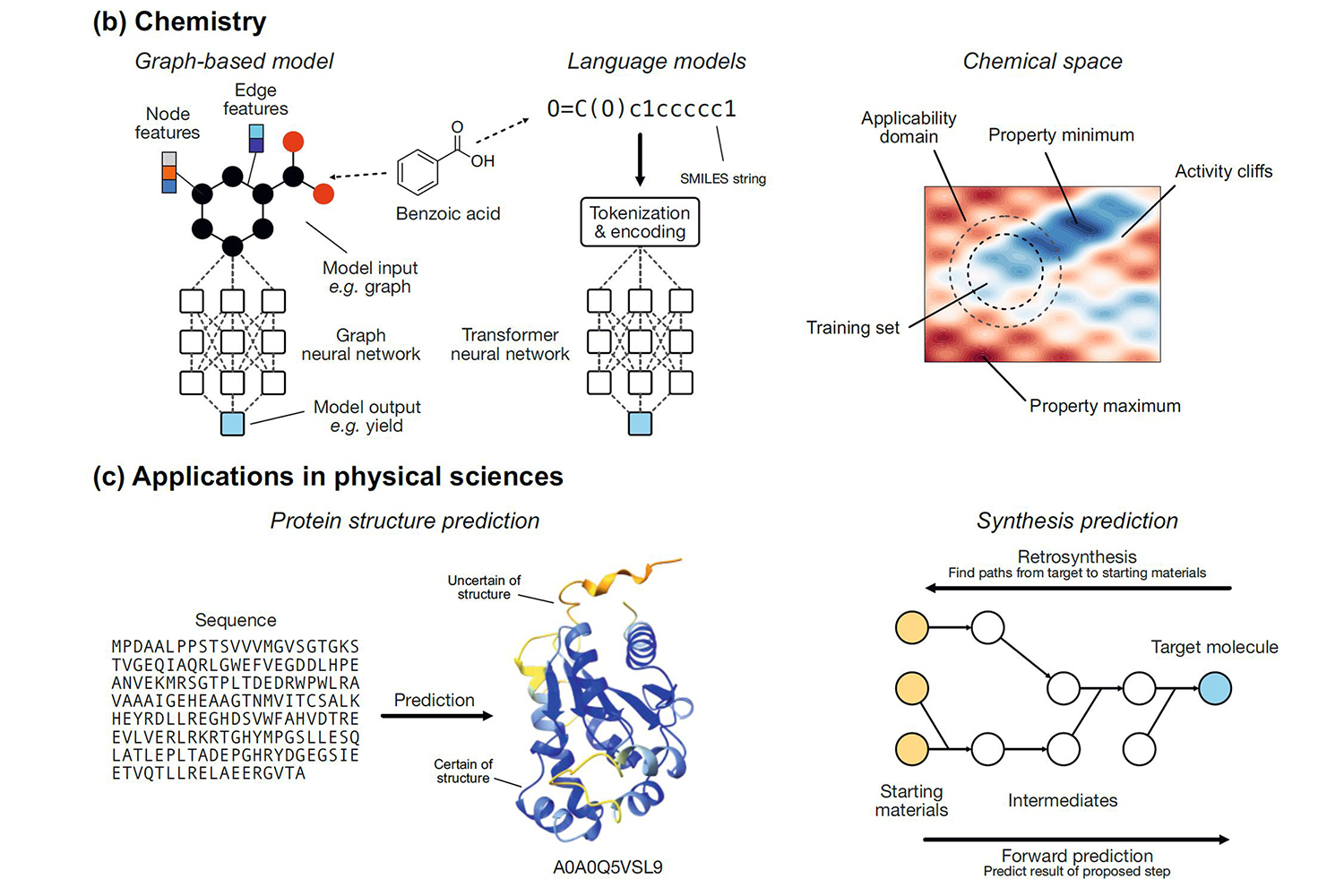
ML systems need to be trained on as much data as possible for optimal performance. In chemistry, however, large datasets are available only in a few areas – e.g., for retrosynthesis models (trained with about 3.3 million reactions) – and even there, the data sets are worlds smaller than those of image systems (DALL-E: about 250 million image-text pairs) or text systems (ChatGPT 3.5: about 500 billion tokens). "Another problem in chemistry is that the data is usually not available in the right format," Jorner points out. "There are also inevitable differences in data quality between research areas." This makes modeling more challenging.
Getting a lot out of little data
Researchers are trying to enrich the data sets with more information using special methods to inform the computer. "If you want to teach it what a molecule is like, you can do that, for instance, by using descriptors: numbers that describe certain properties of the molecule, such as ionization potential, electron affinity, and so on," Jorner explains. "However, creating descriptors requires special knowledge; not everyone can do it. That is why some catalogs have been created for important groups of molecules. I myself was involved in a catalog for phosphine ligands, which play an important role in catalysis."
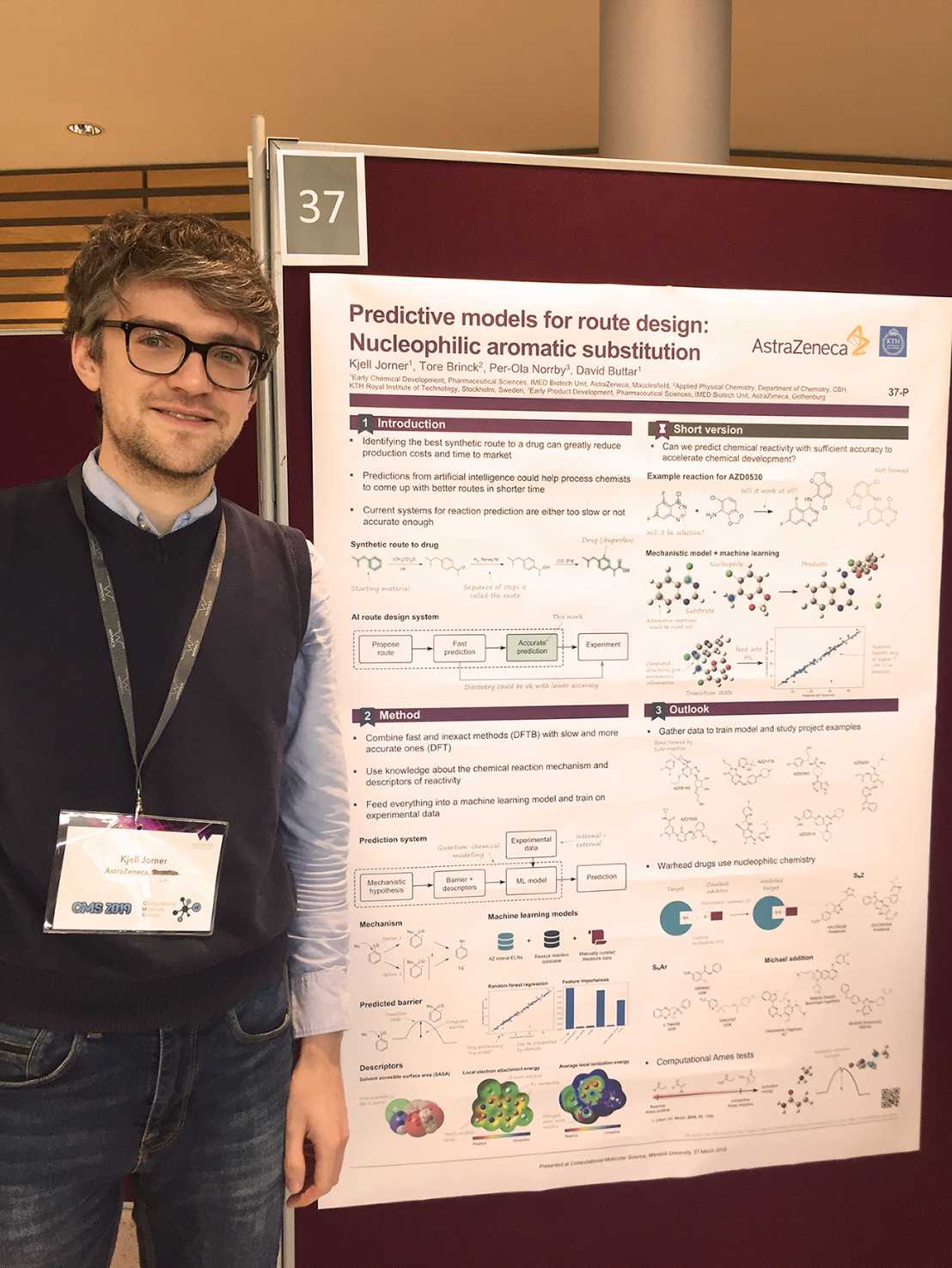
So-called hybrid models, which combine physical simulations with machine learning, can also help to achieve better accuracy when data volumes are low. This is the path that Kjell Jorner took in 2018 as a postdoctoral researcher at AstraZeneca in the United Kingdom. "To make the active ingredient for a drug, you have to build the molecule step by step. Our task was to develop computational tools that could predict whether the steps would be successful and whether they would be selective enough to produce a clean product rather than a mixture that would be costly to purify."
Jorner and his colleagues took a hybrid approach (Jorner et al 2021), choosing the nucleophilic aromatic substitution reaction as a target for application: "We mapped the steps of the chemical reaction with a mechanistic simulation model and complemented this model with AI support using descriptors to achieve higher prediction accuracy." The idea proved successful: the model was eventually adopted by AstraZeneca and other pharmaceutical companies such as Pfizer.
Sustainable chemical production through AI
In 2021, Jorner moved to the University of Toronto for a second postdoctoral fellowship, where he investigated the molecular design of catalysts and organic electronic materials – knowledge that now serves him well as an Assistant Professor of Digital Chemistry at the Chemical and Bioengineering Institute (ICB) at D-CHAB, ETH Zurich. As part of the D-CHAB-associated NCCR Catalysis, he and his group will research digital tools that accelerate the discovery of new catalysts, improve existing catalysts, and optimize experimental conditions. This should help, for example, to find substitutes for fossil fuels as raw materials for chemical production, to develop more sustainable methods for working with fine chemicals, and to enable the production of chemicals from biomass.
He is also planning an elective course on digital chemistry and is looking forward to mentoring students as his group grows. In addition, exciting times lie ahead for the university. "Right now, researchers, teachers, and leadership at ETH are all having lively discussions about how to use AI tools. Everything is changing so fast, and people are struggling to keep up. While a ban on AI seems infeasible," Jorner muses, "we should equip our students with the knowledge to use these tools properly since these skills will become essential for the job market. However, the widescale use of AI necessitates that we adapt our teaching and assessing methods."
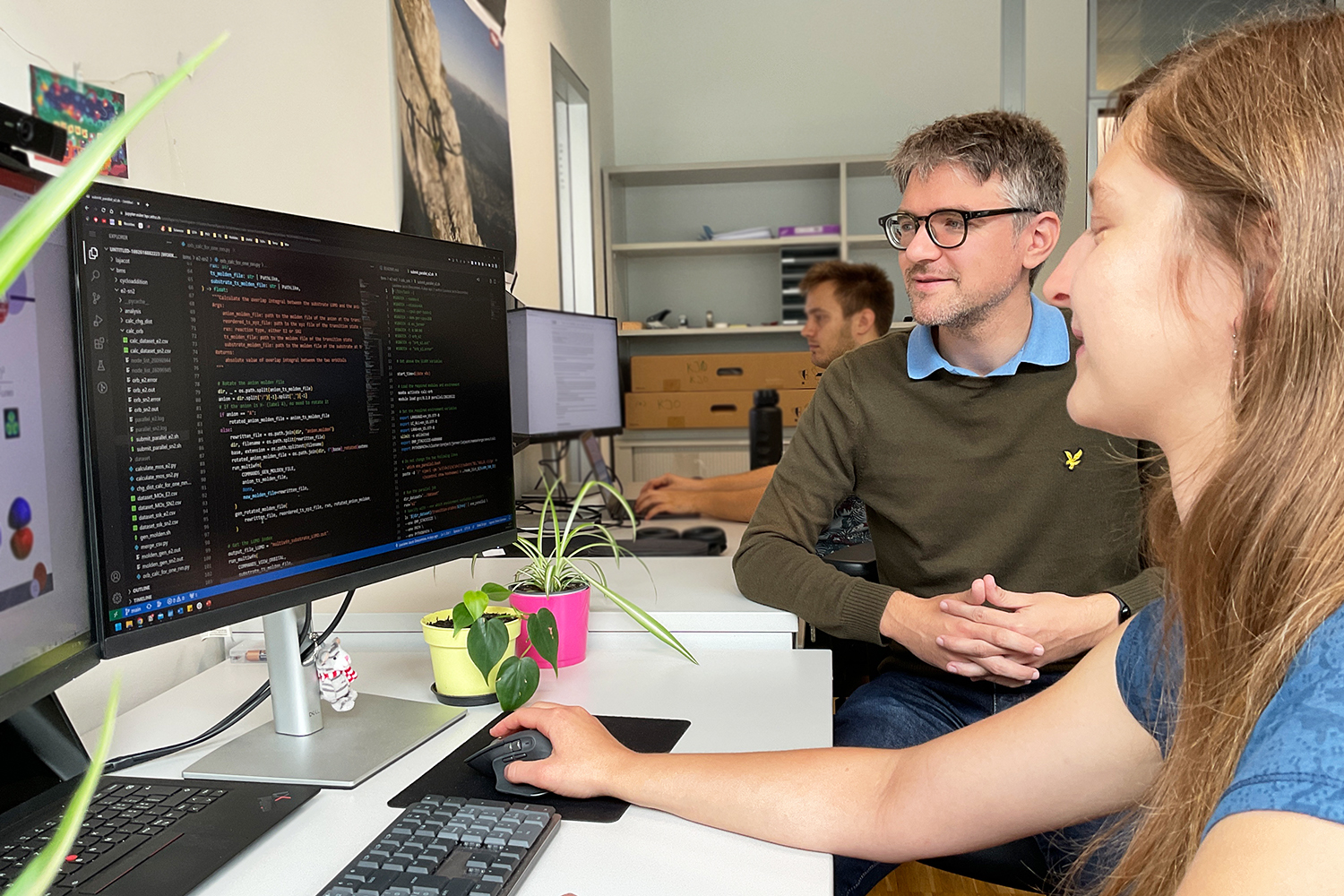
Ultimately, the consequences of these developments are very difficult to assess, Jorner admits: "In fact, some prominent voices are calling for a moratorium on AI research or strict regulations that would limit AI research to large companies. But it will be difficult to stop the development. I agree with the view of the ETH AI Center that it is better to continue open and transparent academic research. Otherwise, others will move forward anyway, and we will have less transparency."
Indeed, AI has great potential. "Our vision would be a system that could design catalysts with suitable properties in response to a user request. Then you could test and synthesize them directly." In a fully automated lab, for example? "That’s probably where the development will go," the professor suggests. Prototypes already exist. But no need for hasty excitement – a lot of coffee will still be flowing through the world's espresso machines, including Kjell Jorner's moka pot, before we get close to practical implementation.
Further information
Jorner K, Brinck T., Norrby PO, Buttar D (2021): Machine learning meets mechanistic modelling for accurate prediction of experimental activation energies. Chem. Sci., 2021, 12, 1163. DOI: external pagehttps://doi.org/10.1039/D0SC04896H
Jorner K (2023): Putting Chemical Knowledge to Work in Machine Learning for Reactivity. Chimia 2023, 77, 22, DOI: external page10.2533/chimia.2023.22.